In the past, we founders have seen various business transformation initiatives at close range. We’ve seen how large companies made strategic business decisions based on rigorous investigation, evaluation and controlled experimentation.
Over the years, we’ve also seen that most companies tend to optimize their [business] strategy and numbers using standard tools. But standard tools (like the usual performance management tools) don’t lend themselves to non-standard ‘resources’ – like employees.
In fact the very use of the term ‘resource’ belies the fact that employees are individuals, unique in every way, even in the workplace where they-
- Perform different jobs
- Perform under different motivations (so that no two people work alike or for the same reasons)
- Don’t always work rationally (even though it is easy to assume that they do)
Behavior Science research and our experience had shown us that an employee’s work is a result of what drives them – whether it be internal motivation, incentives or any other conditions that may trigger them. However, performance management tools don’t adequately capture these motivational factors and hence don’t really impact performance to the degree that they should.
worxogo’s personalized performance coach powered by AI
Based on this lacuna, we launched our own strategic experiment 5 years ago with our AI based performance coach that
- Uses technology and human sciences to understand behavior and motivation
- Captures employees’ work behaviors and triggers
- Connects and clarifies behavior-performance gaps, and
- Delivers personalized, real time nudges and feedback that enables employees to succeed at work
We did not want the tool rely only on academic studies, typically done on small, homogenous samples, in simulated conditions.
To avoid blind alleys, we realized the need for our product to be based on deep explorations and experimentation.
Every company experiments. From piloting new features to A/B testing marketing copy, systematic experimentation drives good, solid decisions and informs long term strategies. But success depends on controlling the variables you test, the conditions in which you test and the tools and frameworks you use to run and manage org-wide experiments.
Thanks to the advancements in data processing and storage, we could provide AI with a large number of data instances to learn from real work situations. By analyzing employees’ behaviors as they interacted with our engine, the AI engine could improve its interventions based on how people responded. In 2017, worxogo’s Behavior Labs took charge of designing and deploying behavior science-based experiments on these data sets through our AI-engine.
Makings of behavior design experiments that impact performance
Among the earliest behavior-performance experiments our engine ran, there was one where we explored Robert B Cialdini’s universal principles of behavior change. We examined ‘Commitment and Consistency’ – one of the six principles, which hypothesized that people’s actions tend to be consistent with the commitments they make – especially when those commitments are made publicly.
The primary research for this came from a study done by Thomas Moriarty on a beach in New York.
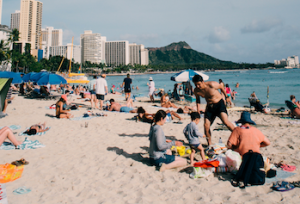
Moriarty’s study sought to establish if active and public commitment led to bystanders intervening in a staged theft. When fellow beachgoers were mere bystanders, they were quite happy to let a ‘thief’ make off with someone else’s portable radio. But under conditions of commitment (when the fellow beachgoer agreed to watch that person’s things) “the results were clearly reversed and 95% of the bystanders were responsive and even intervened in the theft,” the study reported. The sample size of the study was 56, but this was an important finding that was later used to design governmental anti-crime policies.
We explored how this ‘commitment and consistency’ principle could trigger better performance in an industry context.
A factory of a large Indian multinational conglomerate we worked with wanted to reduce vendor delivery delays to enhance their customers’ experience. Using our findings around active and public commitment, the engine helped roll out an updated delivery commitments module to more than 1000 of their vendors using the company’s native software.
Typically, this software would send out vendor requests with details of the part needed, quantity required, and date by which it was due. Our application, which sat on top of the company software – provided three options to the ‘due date’ field – the first was to ‘agree’ to the set date of delivery, the second was to ‘disagree’ and commit to an alternate date, and the third was to ‘leave it as is’, without any changes. The behavior response as well as outcomes were mapped by the AI engine.
We soon found that vendors who had actively committed to the set date performed 2.5 times better at delivering on time than those that left the date as is. The commitment consistency principle was true in workplaces and impacted performance. But more importantly, it proved that by focussing on the ‘why’, our behavior sciences-based performance tool was able to bring about positive behavior changes in a short period of time.
Making the switch: Optimizing performance using behavior sciences
Over the years, worxogo’s Behavior Labs, through our AI engine, has tested several behavior theories, investigating what really drives people at work, what makes them successful and what unique factors trigger each individual. These findings are fed back into our AI-ML nudge engine making it a one-of-a-kind learning digital performance coach.
In addition to this, these behavior insights offer a unique behind-the-scenes glimpse into teams – helping leaders make better team decisions and roll out effective people strategies.
I’m excited that we’re at the helm of this behavior-led performance journey with our customers. You can read more of our customer stories here.
Photo by Ryoji Iwata on Unsplash